Based on the Formula Student Driverless (FSD) competition, this thesis is devoted to the construction of a scale-down electric vehicle, and the self-driving system for the vehicle to autonomously run on the track defined by traffic cones. The design of the electric vehicle follows the requirements of the FSD competition. Its propulsion force is provided by four brushless DC motors installed on each of the wheels, and the steering is accomplished by two small servo motors that independently orient the two front wheels. In addition, the vehicle is also equipped with an optical camera, an optical-flow speed sensor, accelerometers, and gyroscopes. The self-driving system contains two parts: sensor fusion and navigation control. The sensor fusion part uses Extended Kalman Filtering and FastSALM-1.0 algorithm to fuse camera and various sensor information to estimate vehicle location and cone map information. Particularly, the cone map information can be further processed using Delaunay triangulation so as to recover the path area of the track. The navigation control part utilizes a graphic processor unit to simulate vehicle dynamics for a massive number of control input trajectories parallelly, and then applies stochastic optimization to solve model predictive control so that control inputs achieving both attitude stability and tracking performance can be obtained. Through simulations and experiments, it is proved that the proposed methods can accomplish the real-time exploration and self-driving on the racing track under unknown-map condition.
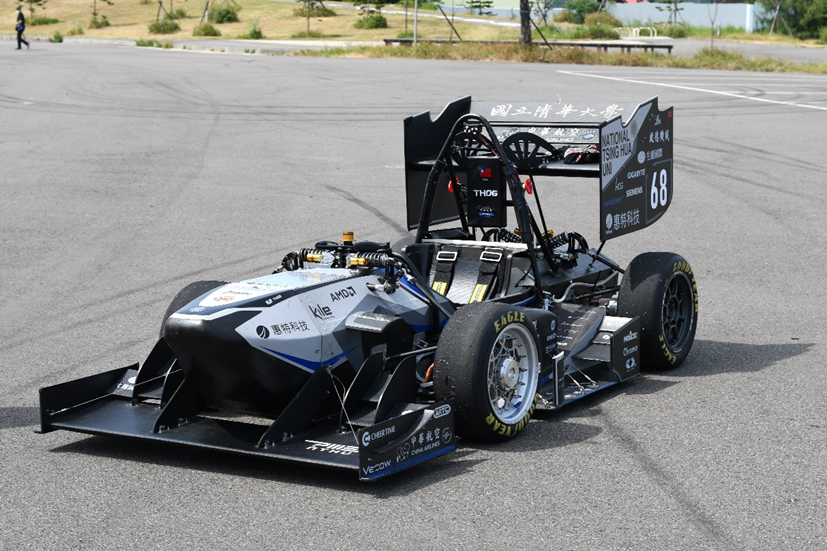
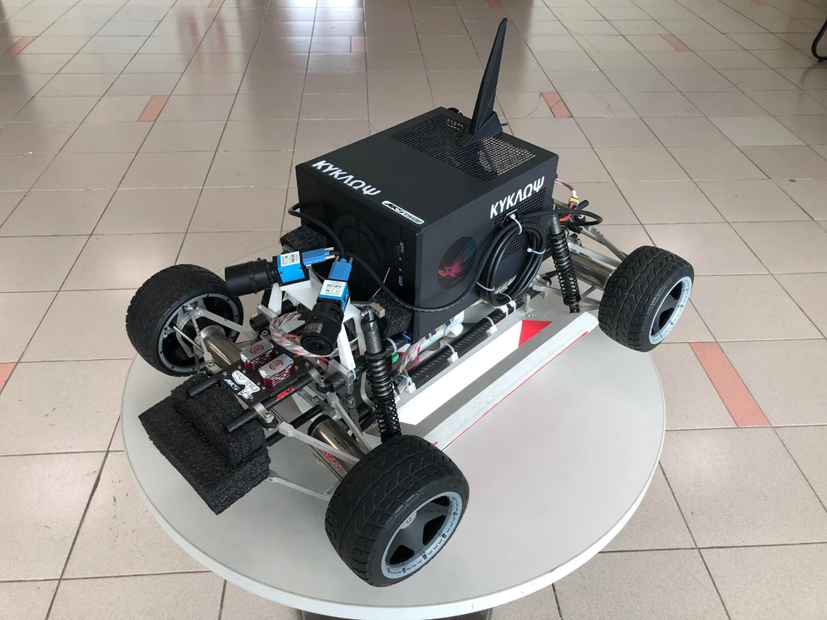